
The original intent was to turn this documentation into a full tutorial series similar to the Stylized Character Workflow. The main topic will also be on the sculpting and modeling process for animation, instead of the whole workflow of texturing, shading, grooming, rigging and animation. It will not go into detail like other tutorials on this platform. This documentation is focused on advanced techniques and general advice that was learned during production. This documentation will remain more generalised to apply to other characters outside of that production as well. Based on actual movie productionĪll the content is closely tied to Project Heist and will often use it as a visual reference. This section was mostly compiled from previous work and research from the Blender Studio. We demonstrate that our approach significantly outperforms existing state-of-the-art techniques on single image human shape reconstruction by fully leveraging 1k-resolution input images.In this documentation we share all the current research and workflow techniques on creating highly realistic human characters in Blender.

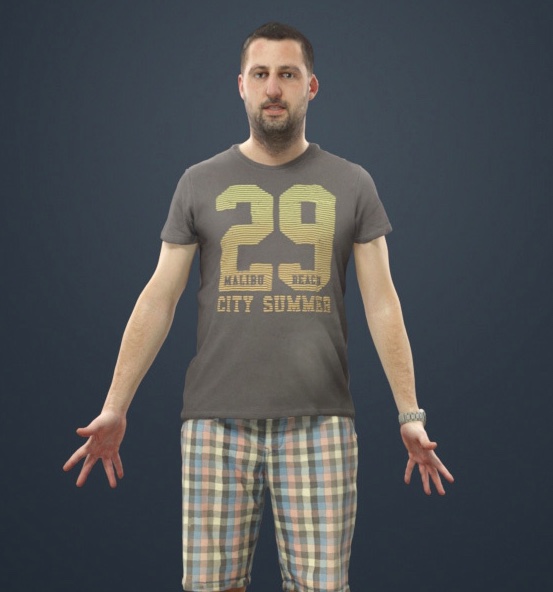
This provides context to an fine level which estimates highly detailed geometry by observing higher-resolution images. A coarse level observes the whole image at lower resolution and focuses on holistic reasoning. We address this limitation by formulating a multi-level architecture that is end-to-end trainable. Due to memory limitations in current hardware, previous approaches tend to take low resolution images as input to cover large spatial context, and produce less precise (or low resolution) 3D estimates as a result. We argue that this limitation stems primarily form two conflicting requirements accurate predictions require large context, but precise predictions require high resolution. Although current approaches have demonstrated the potential in real world settings, they still fail to produce reconstructions with the level of detail often present in the input images. Recent advances in image-based 3D human shape estimation have been driven by the significant improvement in representation power afforded by deep neural networks.
